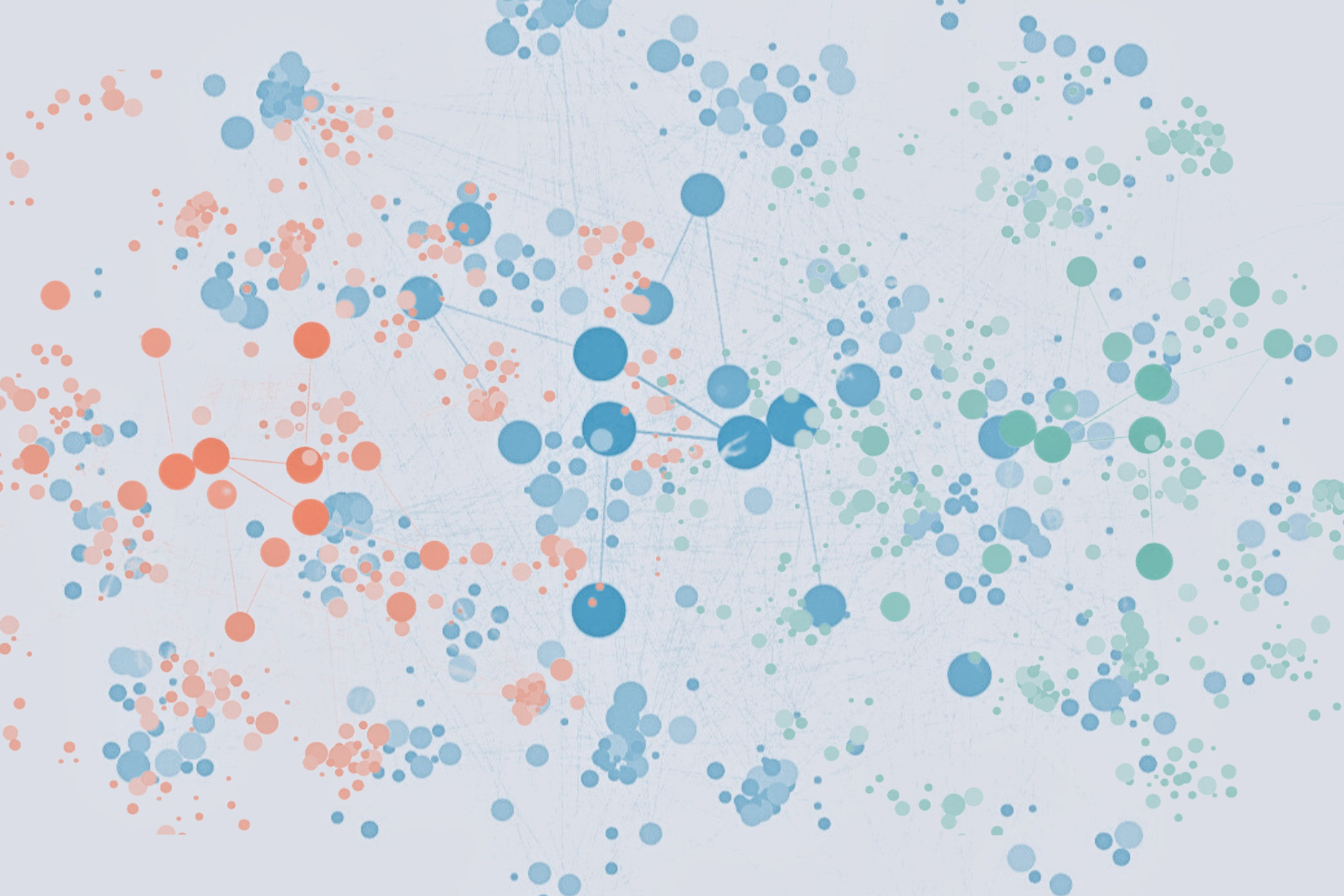
White Papers
Hidden Bias in Hiring
Learning Collider’s inaugural white paper examines applicant screening technologies, drawing insights from Peter Bergman and co-authors’ working paper Hiring as Exploration (available from the National Bureau of Economic Research).
Open the link to see how we connected the dots from research to ever-present challenges facing recruiters and talent acquisition at large.
Algorithms in hiring can be complex. Our white paper breaks down the technical jargon to show how these technologies can and must be made better.
Executive Summary
Hiring algorithms are not new. These gatekeepers are here to stay. What’s needed now is a deeper, collective understanding of how they work - from development to decision-making.
Hidden Bias in Hiring examines machine-learning algorithms used to screen applicants in the early phases of the hiring funnel. We begin with a foundational understanding of hiring algorithms, how they function, how they are trained, and why it matters. We then delve into opportunities to improve algorithmic hiring technology.
This white paper draws from collaborative academic research leveraging real-world data from an anonymous Fortune 500 company.
A team of researchers - including Learning Collider’s founder - initiated their study by building and testing a typical screening tool. What they found wasn’t surprising: standard machine-learning algorithms are problematic in their design, training, and outputs. Instead of their perceived objectivity, this commonplace tool codifies the bias and blindspots of antecedent human decision-makers.
By dissecting the inner workings of the standard model, researchers identified opportunities to improve the algorithmic design, training, and subsequent decision-making. Their next phase was developing and testing an inclusive machine-learning algorithm.
Through simulations with data from roughly 89,000 job applications, the inclusive model outperformed the standard model and human recruiters, selecting more diverse and high-quality applicants for interviews. The estimated savings, given boosted hiring rates, is more than 2/3 of the total interviewing cost (from $1.1m down to $337,000 for 445 offers).
Inclusive machine-learning algorithms have the potential to transform applicant screening by retaining the cost-efficiency benefits of technology while systematically improving hiring decisions and advancing economic mobility for underrepresented applicants.